Anatomy of Artificial Intelligence in the Insurance Value Chain
Director of Operations
(Director of IT Technology and Systems until March 2024)
Introduction
Artificial intelligence (AI) is revolutionising the insurance industry’s value chain in a number of different ways by improving efficiency, accuracy, and the customer experience. The main practical applications and the impact of AI on different stages of the value chain are briefly discussed below:
-
Product development and pricing
- Personalisation: AI enables insurers to offer personalised products based on the behaviour and needs of individual customers.
- Dynamic pricing: insurers can use advanced algorithms and continuously updated data to set prices in real time.
-
Marketing and distribution
- Customer segmentation: AI uses data analysis to identify customer segments and direct marketing efforts most effectively.
- Intelligent sales channels: chatbots and virtual assistants enhance interaction with customers and boost policy sales.
-
Risk assessment and underwriting
- Underwriting automation: AI can quickly process large volumes of data to assess risks and take underwriting decisions.
- Enhanced risk selection: predictive algorithms and deep machine learning models can identify patterns and correlations for enhanced risk assessment.
-
Services and operations
- Internal processes: AI is used to computerise such low-risk internal processes as customer analysis and back-office operations.
- Operational efficiency: AI improves operational efficiency in performing repetitive tasks and data processing.
-
Claims management
- Claims process automation: AI expedites the claims handling process from initial submission to final settlement.
- Fraud detection: AI systems analyse patterns to prevent and detect fraudulent claims.
-
Customer service
- 24/7 support: virtual assistants provide continuous personalised customer support.
- Feedback and continuous improvement: AI compiles and analyses feedback to continuously improve services.
-
Risk management and compliance
- Risk assessment: AI improves the accuracy of risk assessment and portfolio management.
- Regulatory compliance: AI performs data analysis and report generation to help insurers comply with complex regulations.
-
Cybersecurity and data privacy
- Data protection: AI improves cybersecurity by monitoring and detecting threats.
- Data governance: insurers use AI governance models to manage data privacy and security.
In short, AI is transforming every aspect of the insurance industry value chain from product personalisation to claims handling and customer service. As the technology advances, it is expected that it will be integrated further and deeper within the insurance sector, resulting in heightened efficiency and enhanced results for insurance companies and their customers.
This article presents the results of a study (1)2023 Gartner CIO and Technology Executive Survey. analysing more than 500 use cases from insurers and reinsurers around the world, considering three different aspects: the technologies employed, the intended purposes, and the business processes concerned. Relying on more than one technology, for more than one purpose, impacting more than one business process is a feature common to two-thirds of the 585 use cases reviewed.
Artificial intelligence applied to the use cases considered
Artificial intelligence has worked its way into the lives of organisations and of nearly all people and has entered into our day-to-day conversations. If we were to ask anyone what he or she thinks AI is, we would most likely get a different answer from everyone we asked.
This article uses the definition given by the OECD: “An AI system is a machine-based system that, for explicit or implicit objectives, infers, from the input it receives, how to generate outputs such as predictions, content, recommendations, or decisions that can influence physical or virtual environments. Different AI systems vary in their levels of autonomy and adaptiveness after deployment”.
The subject matter normally falling within the scope of the term “AI” includes technical categories like machine learning and knowledge-based approaches and application areas such as computer vision, natural language processing, speech and voice recognition, intelligent decision-making support systems, intelligent robotic systems, and novel applications of these tools in a variety of domains. AI technologies are developing at a rapid pace, and more techniques and applications are likely to emerge in the future. The OECD definition tries to be flexible and is based on a broad understanding of what AI is, and people using this definition are encouraged to assess the relevant scope depending on the context involved.
The use cases considered here are public and cover companies of all kinds in geographic areas spanning all continents for the years 2015 to 2023 (both included).
Globally, non-life insurers account for nearly 69% of the cases and life insurers 28%, the remaining 3% are reinsurers use cases. However, the following table shows that this distribution changes appreciably when broken down into the three different geographic regions in which these use cases fall.
AREA | NON-LIFE | LIFE | REINSURANCE |
---|---|---|---|
AMERICAS | 81.4% | 17.5% | 1.1% |
APAC | 47.0% | 51.4% | 1.7% |
EMEA | 71.6% | 20.5% | 7.9% |
Overall | 68.8% | 28.0% | 3.3% |
Table 1.
Source: 2023 Gartner CIO and Technology Executive Survey.
Non-life insurers are more active in the Americas and EMEA (2)Europe, Middle East, and Africa. regions, whereas life insurers are slightly more active in the Asia Pacific region (APAC).
Technologies in use
As mentioned above, the definition of AI covers a broad range of very different technologies used for a wide variety of purposes. The technologies used in the cases considered here are discussed below, briefly describing each technology and outlining how they are used. A chart showing the intended purposes and the business processes concerned is included for each of the use cases.
A. Chatbot
A chatbot is computer software that uses artificial intelligence to simulate conversations with users. Responses can be automated and customers guided through, e.g., sales, reservation, claims, and product return procedures. Chatbots can learn and can adapt to the context and to user needs, making it easier to search information without human involvement. Using this technology optimises communication, enhances the customer experience, and helps insurers provide more efficient services by providing:
- Efficient communication: chatbots enable customers to complete insurance procedures quickly and securely. They can also serve as a channel for collecting data on customer behaviour, preferences, and needs.
- Cost reduction: by being able to connect with multiple customers at once, insurers can lower marketing costs and achieve more effective results.
- Support services for the insured: chatbots can provide entry-level support for such questions and incidents as finding a loss adjuster, obtaining information about renewals or expiry dates, and contacting brokers.
The following chart presents a summary of the intended goals of insurers and the internal processes involved for the use cases considered.
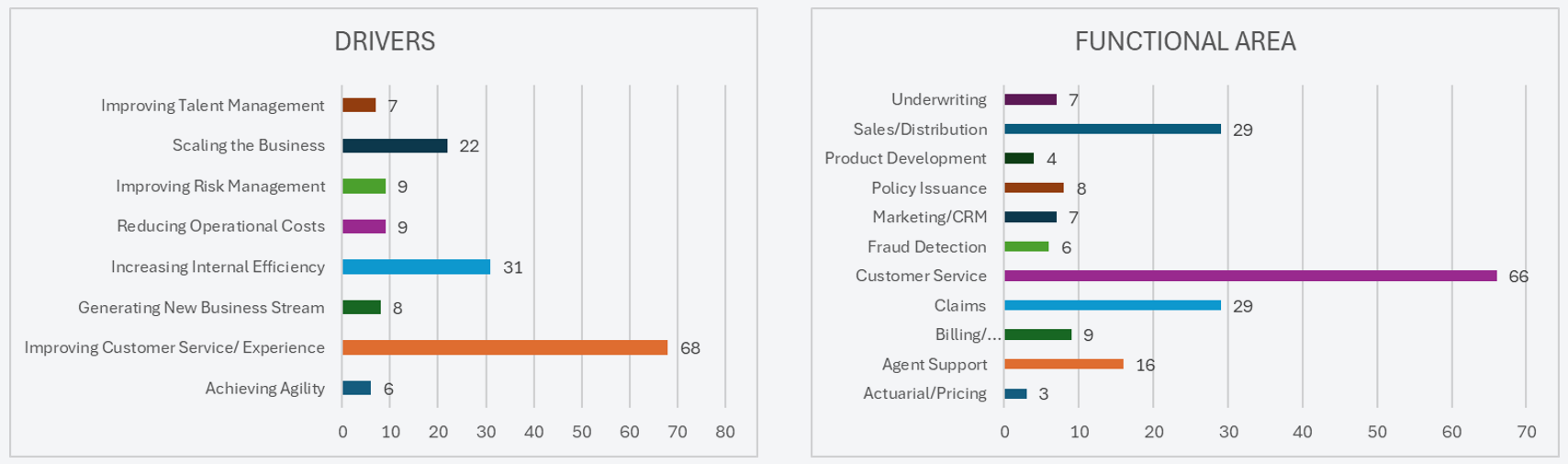
Figure 1.
Source: 2023 Gartner CIO and Technology Executive Survey.
B. Deep neural networks
Deep neural networks are computational models patterned on the neural structure of the human brain. They consist of layers of interconnected artificial neurons designed for distributed hierarchical data processing. In other words, these networks use a series of layers to learn and extract increasingly complex abstract characteristics as we move deeper into the network. They can be used for applications ranging from fraud detection in the insurance sector to computerising tasks that are otherwise hard to do using conventional programming.
Deep neural networks have become a valuable tool within the insurance industry. Some of their applications are:
- Fraud detection: identifying unusual transaction patterns and detecting fraudulent claims. These networks analyse historical data such as the details of incident reports and pay-outs to prevent fraud before any monies are paid.
- Contract migration: modernising conventional systems is critical to insurers. Deep neural networks can help automate the migration of millions of insurance contracts from the source systems to a target system, including actuarial functions for contract management.
- Accuracy and explainability: accuracy needs to be high, but explainability is also required. Neural networks can generate black box models, making it difficult to understand how they achieve their results. This is critical for purposes of complying with industry regulations.
The following chart presents a summary of the intended goals of insurers and the internal processes involved for the use cases considered.
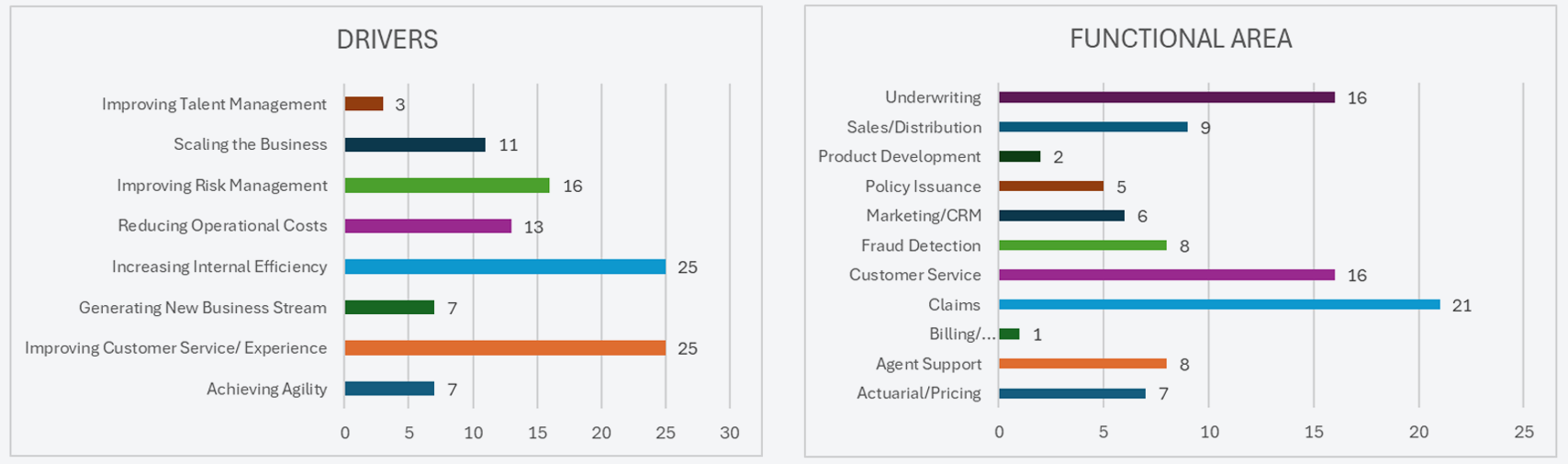
Figure 2.
Source: 2023 Gartner CIO and Technology Executive Survey.
C. Machine learning
Machine learning (ML) is a branch of artificial intelligence that deals with developing algorithms and statistical models that enable computers to perform tasks without being explicitly instructed and instead using patterns and inferences.
ML has turned into a valuable tool within the insurance industry. Its applications include:
- Customer advice and policy sales: insurance companies can use conversational AI to interact with people and provide personalised advice. Cognitive virtual assistants (CVAs) help customers navigate the different coverage options, answer customer questions, and make recommendations based on customer needs and budgets. Policies can even be purchased through a chatbot without having to talk to an employee, speeding up the process and making it easier.
- Claims automation: automating the claims and reimbursement settlement process is another interesting application of conversational AI in the insurance industry. CVAs can collect information about a claim, evaluate whether it is eligible, and provide status updates. This not only reduces the cost of processing claims but also improves productivity and customer satisfaction.
- Data analysis and risk prediction: ML enables insurers to analyse large volumes of data to predict risks and set pricing more precisely. This helps optimise policies and take informed decisions.
The following chart presents a summary of the intended goals of insurers and the internal processes involved for the use cases considered.
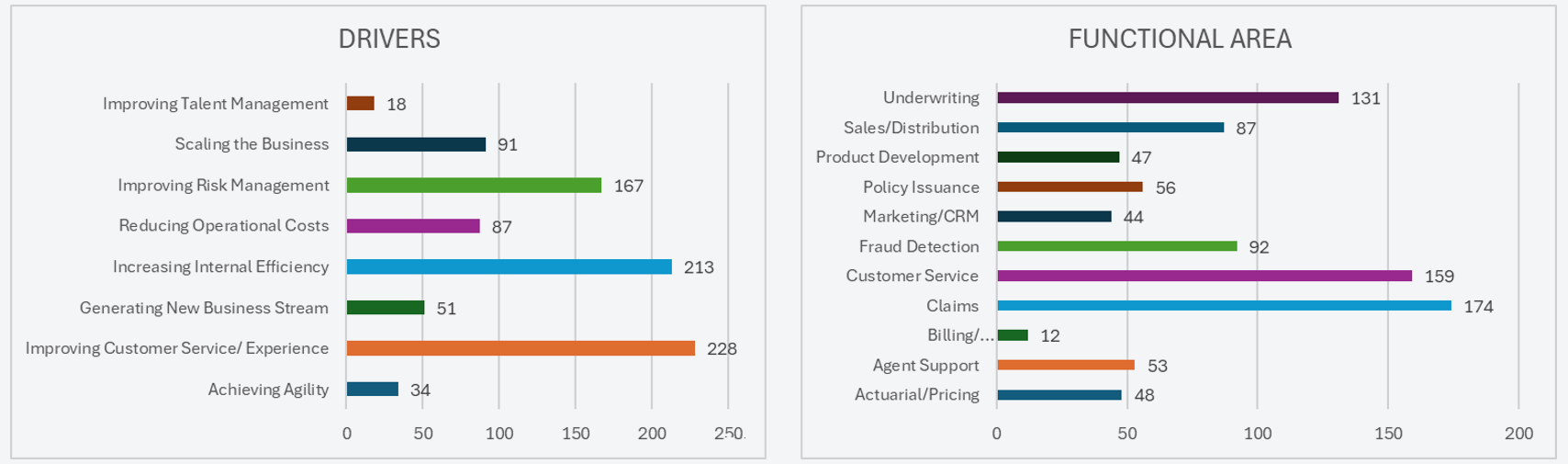
Figure 3.
Source: 2023 Gartner CIO and Technology Executive Survey.
D. Natural language processing
Natural language processing (NLP) is a branch of AI that is at the core of applications and devices capable of:
- Translating text from one language to another.
- Responding to spoken and written orders.
- Recognising or authenticating users by their voice.
- Summarising large volumes of text.
- Evaluating intent or sentiment in a text or speech.
- Generating graphic or text content on demand, often in real time.
In short, NLP enables computers to understand and generate text and speech and has applications from voice-operated GPS systems to customer attention chatbots and business solutions to automate operations and simplify critical processes.
NLP has been transforming the insurance industry for years by being used in:
- Claims management: AI platforms and NLP expedite claims processing by increasing accuracy and coherence. For example, after implementing an AI system, Zurich Seguros decreased the time spent on reviewing claims by 58%.
- Input processing: insurers evaluate a broad spectrum of information daily (medical reports, emails, etc.). NLP enables these documents to be organised automatically, saving time and improving coherence. Generali uses NLP to read, interpret, and distribute over a million emails a year.
- Policy review: NLP helps ensure that policies are suitable and limits exposure to risks ahead of underwriting. Its use in automation improves efficiency and consistency.
The following chart presents a summary of the intended goals of insurers and the internal processes involved for the use cases considered.
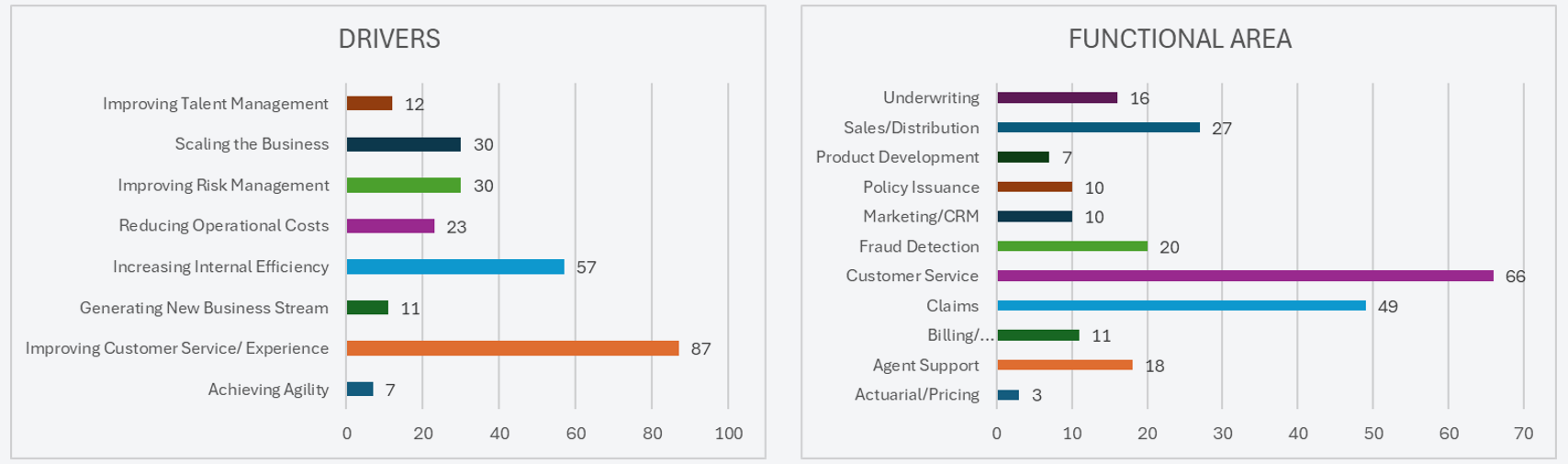
Figure 4.
Source: 2023 Gartner CIO and Technology Executive Survey.
E. Robotic process automation
Robotic process automation (RPA) is a software technology for building, implementing, and managing virtual robots that emulate human actions when interacting with software and digital systems. These “robots” can carry out repetitive tasks like extracting data, filling out forms, moving files, etc. Unlike people, robots do not need to take breaks and can perform tasks quickly and consistently.
Some of the main use cases are:
- Claims processing: expediting claims processing by decreasing times and reducing manual handling. Claims can be processed 75% faster than by hand.
- Underwriting: automating risk assessment and policy approval.
- Regulatory compliance: contributing to regulatory and legal compliance by computerising such tasks as document verification and monitoring changes in the law.
- Sales and distribution: automating the processes of providing quotes, issuing policies, and effecting renewals.
- Finance and billing: managing accounts receivable, accounting sources, ledgers, and account closure.
- Policy management: automating the management of changes to policies and data updates.
- Business and process analysis: helping with identifying areas for improvement and optimisation.
- Legacy applications: integrating former systems and eliminating organisational silos.
The following chart presents a summary of the intended goals of insurers and the internal processes involved for the use cases considered.
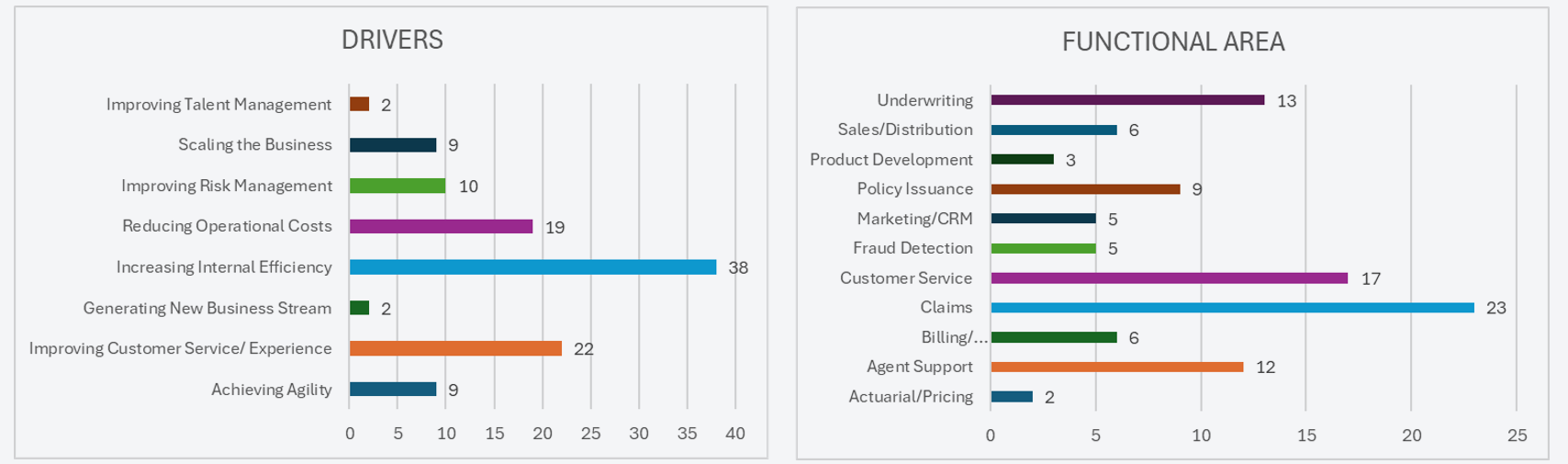
Figure 5.
Source: 2023 Gartner CIO and Technology Executive Survey.
F. Speech recognition and cognitive virtual assistants
Speech recognition technology enables computers and other devices to identify and process spoken human language and convert it to text. It uses computational linguistic methods, computer science, electrical engineering, and similar disciplines to develop systems capable of understanding and translating words and phrases.
There are a series of operational steps:
- Word detection: the system identifies spoken words.
- Hypothesis and testing: different hypotheses about what has been said are generated and compared to a language model to check their consistency.
- Text processing: speech is converted to written text, including such elements as numbers and punctuation marks.
Over time this technology has advanced considerably from the early systems that recognised numbers and simple words to today’s systems that understand natural speech and can be trained to recognise individual voices, thus improving their accuracy.
Cognitive virtual assistants (CVAs) are advanced artificial intelligence systems designed to interact naturally with users either in text or spoken form. They use natural language processing algorithms and machine learning to understand and answer user queries and offer customers an efficient, personalised customer service experience.
Some of the main uses of CVAs are:
- Omnichannel interaction: they operate across multiple platforms and channels such as email, SMS, WhatsApp Business, social media, and others, enabling users to communicate with them using their medium of choice.
- 24/7 availability: they are available around the clock, so companies can offer their customers continuous support.
- Personalisation: they can be trained and aligned with the company’s identity using corporate colours and personalised avatars.
- Integration with transaction services: they allow secure integration with customer transaction services, accessing private data when necessary.
- Data analysis and storage: they keep a record of interactions, enabling companies to analyse and improve service quality on an ongoing basis.
The upshot is that CVAs are a valuable tool for companies looking to implement artificial intelligence solutions to enhance the customer experience and optimise their service processes.
In several use cases CVA and speech recognition technologies have been combined. For instance:
- Customer advice and policy sales: conversational artificial intelligence can be used to interact with people and provide personalised service. Cognitive virtual assistants (CVAs) help customers navigate the different coverage options, answer customer questions, and make recommendations based on customer needs and budgets.
- Claims automation: automating the claims and reimbursement settlement process is another interesting application. CVAs can collect information about a claim, evaluate whether it is eligible, and provide status updates.
- 24/7 customer service: CVAs provide non-stop support, answer questions, and provide clear information every step of the way to guide customers through the various procedures.
- Risk assessment: conversational AI can help assess the risks attaching to insurance policies, improving the process’s efficiency and accuracy.
- Fraud detection: speech recognition systems are capable of analysing conversation patterns and detecting words and phrases commonly used in frauds. In addition, AI can even use voice recognition to verify customer identity so as to prevent identity theft.
The following chart presents a summary of the intended goals of insurers and the internal processes involved for the use cases considered.
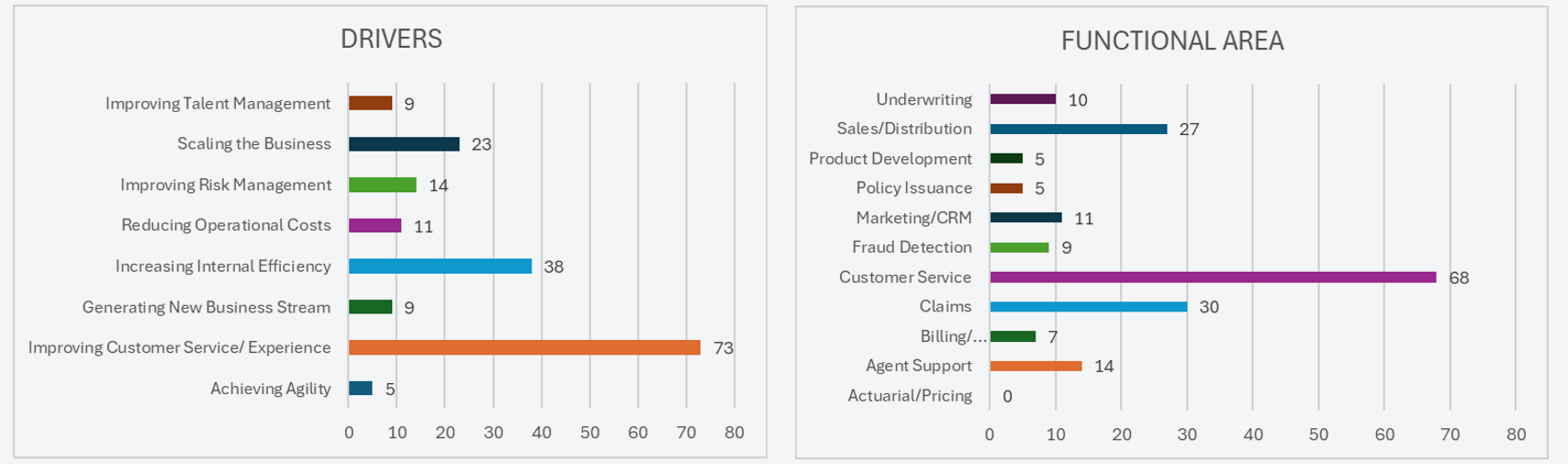
Figure 6.
Source: 2023 Gartner CIO and Technology Executive Survey.
G. Generative AI
Generative artificial intelligence is a technology that creates new content based on deep learning models that have been trained on massive datasets. These models differ from discriminative AI models, which are used to classify data based on their differences.
It has three main phases of operation:
- Training: a foundation model that can be used as a basis for a variety of generative AI applications is created.
- Tuning: the foundation model is tailored to a specific generative AI application.
- Generation, evaluation, and retuning: the generative AI application’s output is evaluated and its quality and accuracy are continuously improved.
Generative AI is used to generate texts, images, code, and much more and has practical applications in chatbots, in image creation and editing, in writing software code, and in scientific research. It is also used in professional settings for fast creative idea visualisation and efficient execution of monotonous, time-consuming tasks.
Some of the best-known generative AI applications are OpenAI’s ChatGPT and DALL-E, GitHub CoPilot, Bing, Microsoft Chat, Google Bard, Midjourney, Stable Diffusion, and Adobe Firefly. These tools are revolutionising how we interact with technology and how routine tasks are automated.
Generative AI has found numerous applications in the insurance sector and is transforming how companies operate and relate to their customers. Some use cases include:
- Process automation: generative AI can automate repetitive tasks like analysing and writing summaries of documents, enabling insurers to process claims and policies more efficiently.
- Analysing large quantities of data: with its ability to analyse large volumes of data, generative AI can help insurers gain valuable insights about customers and markets.
- Customer support automation: chatbots powered by generative AI can interact with customers, answer their questions, and guide them through complicated procedures, enhancing the customer experience.
- Managing and reading customer emails: generative AI can be used to manage and read emails to identify customer needs and generate personalised responses.
- Damage detection through vehicle image analysis: when insurance is being purchased, generative AI can analyse images of vehicles to detect damage, thereby aiding in risk assessment.
- Automated content creation: this technology can generate content for digital platforms, such as social media articles and texts, helping to improve search engine positioning and interaction with users.
- Claims management optimisation: generative AI can automate claims processing from the initial evaluation to final decision-making, speeding up the process and lowering operating costs.
The following chart presents a summary of the intended goals of insurers and the internal processes involved for the use cases considered.
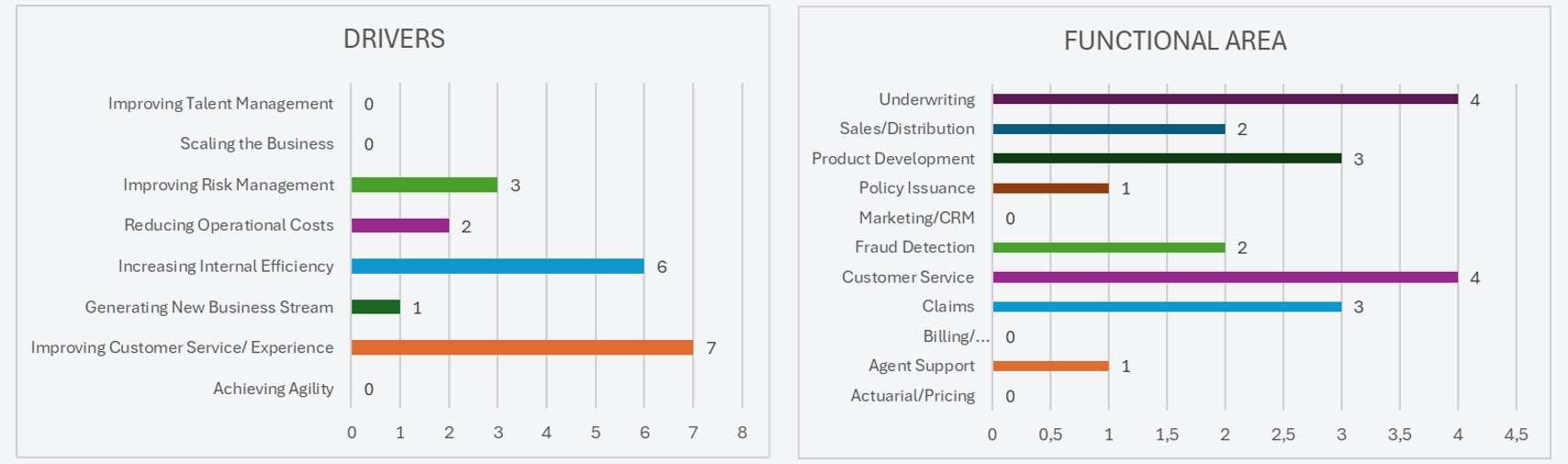
Figure 7.
Source: 2023 Gartner CIO and Technology Executive Survey.
H. Face and image recognition
Face recognition is a biometric technology used to identify or verify the identity of an individual from the face. It is used to recognise people in photographs, videos, or even in real time. It includes:
- Face detection: the camera detects and locates the image of a face, either alone or in a crowd.
- Face analysis: the image of the face is captured and analysed. Most face recognition technologies are based on 2D images, which can be more readily compared to public photographs or photographs in databases.
- Image to data conversion: the captured face is converted to a digital dataset based on the individual’s facial features. This numeric code is called the facial footprint and is unique to each individual, like fingerprints.
Image recognition is a broader technology that is not restricted to the human face. It uses computer vision algorithms to detect, process, and classify data gleaned from images. It can identify objects, places, individuals, writing, and actions in images and videos.
There are several use cases of face and image recognition by the insurance industry. It can improve security and efficiency considerably:
- Identity verification: face recognition can be used to verify the identity of customers during the policy application or claim submission process to prevent fraud.
- Claims processing: image recognition can be used to computerise the review of visual documents like photographs of automobile accidents. This speeds up the claims process and lessens the chance of human error.
- Risk assessment: image recognition can help assess risk by analysing images of properties or vehicles to detect features that could indicate a higher risk of loss.
- Personalised services: by using image recognition insurers can offer personalised services based on customer preferences and behaviour detected by means of image analysis.
The following chart presents a summary of the intended goals of insurers and the internal processes involved for the use cases considered.
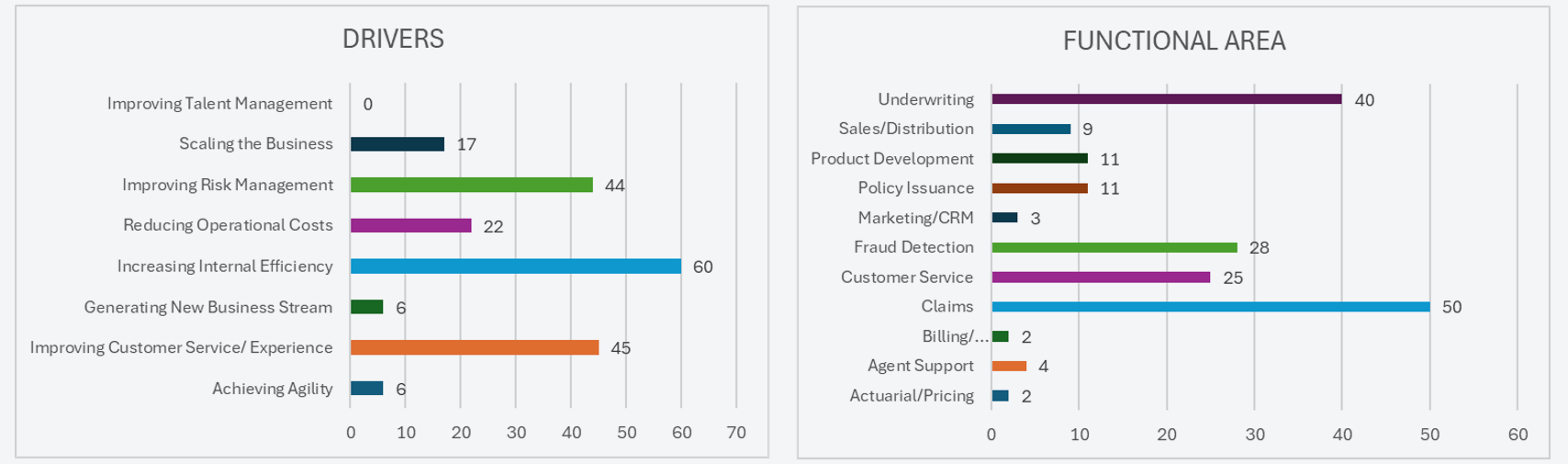
Figure 8.
Source: 2023 Gartner CIO and Technology Executive Survey.
This article uses the definition given by the OECD: "An AI system is a machine-based system that, for explicit or implicit objectives, infers, from the input it receives, how to generate outputs such as predictions, content, recommendations, or decisions that can influence physical or virtual environments. Different AI systems vary in their levels of autonomy and adaptiveness after deployment".